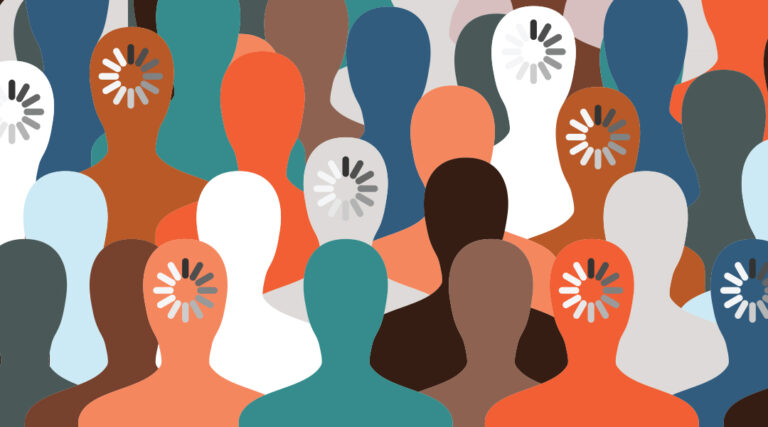
Enterprises are happily launching themselves into the wonderful world of machine learning, without thinking about any particular methodology to guide their efforts an O’Reilly survey of over 11,000 people has revealed.
Likewise, taking account of bias and fairness are some way down the list for organisations just beginning to explore the potential of machine learning technology.
The survey covered 11,400 respondents worldwide, though the vast majority – 53 per cent – were in North America, with Western Europe the next biggest geography at 13 per cent. Just over half the organisations were classed as in the early stages of adopting machine learning, with early adopters (up to two years in) making up 36 per cent and “sophisticated” companies making up the rest.
More experienced outfits were more likely to build their own machine learning models, at 73 per cent. Beginners were the most likely to use external consultants (12 per cent). Likewise the more sophisticated organisations were more likely to use ML-specific job titles – such as data scientist and data engineer.
Just 3 per cent of organisations overall used Cloud-based machine learning services, a figure that dropped to 2 per cent for both sophisticated and early adopter organisations.
With all this talk of “science” and “engineering”, it might be a surprise that a third of respondents admitted to having no methodology to govern the way they approach their projects. Just shy of 50 per cent used Agile, while 9 per cent used Kanban, and 11 per cent used “other” methods.
Less surprisingly, perhaps, the “no methodology” approach was more common at the newbie organisations, O’Reilly found, with just 22 per cent of sophisticated and 24 per cent of early adopters being unencumbered by methodology.
Recent concerns about ethics and bias appear to have made an impact, with 17 per cent of organisations checking for possible bias in their models and including that as one of the metrics of success. Unsurprisingly, business metrics and ML/statistical metrics are vastly more important at 73 per cent and 48 per cent respectively.
Drilling down further, 26 per cent sophisticated and 18 per cent of early adopter orgs used bias and fairness as a metric, compared to 14 per cent of explorer organisations.
Similarly, fairness and bias were cited as part of the model building checklist by 40 per cent of organisations, while privacy was on the list for 43 per cent. Explainability and transparency was part of the checklist for 65 per cent, compliance for 46 per cent and user control over data and models for 45 per cent.
Once again, neophytes were less likely to check for these factors, with fairness and bias making the list for just 32 per cent of explorers and privacy for 39 per cent.
The report’s authors highlight what differentiates the sophisticated ML exponents from their peers, including the use of specialised roles, the building of their own models and the use of “more robust model-building checklists” which include checks for transparency and privacy.
“These points indicate some of the key learnings that derive from deploying machine learning in production, and also where other companies should focus as they begin their journey,” they suggest.
Let’s hope so, as the alternative seems to be a haphazard approach driven by non-specialists, who are, more importantly, untrammeled by concerns over fairness and privacy.