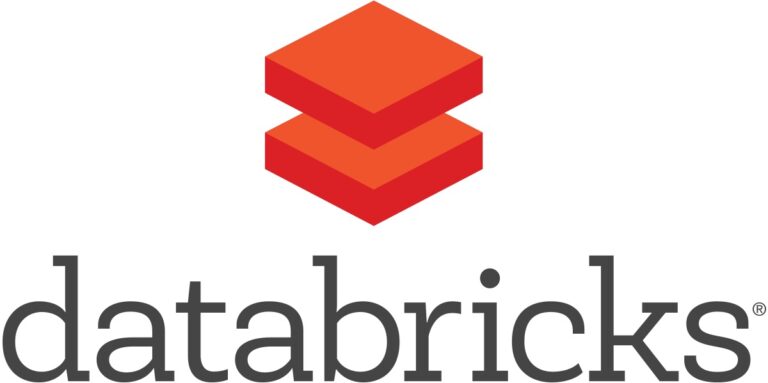
Databricks has announced a public preview of a fully managed version of MLFlow, the machine learning management platform it unveiled last year.
MLFlow is pitched as offering a way to manage the machine learning lifecycle, allowing users to track experiments, package ML code for results, and manage and deploy models. The service is currently in beta, with v0.8.2 shipped at the end of January, and a full fat v1.0 due sometime before the end of Q2 this year.
However, that hasn’t prevented Databricks unwrapping “Managed MLflow”, which it describes as an SaaS version of MLflow with built in management and security, which will integrate MLflow “throughout the Databricks Unified Analytics Platform”.
The debut sees the parent company “embracing MLflow throughout the Databricks Workspace.” For example, it says, “notebook revisions are automatically captured and linked to as part of experiment runs, you can run projects as Databricks jobs, and experiments are integrated with your workspace’s security controls.”
Databricks said it intended to extend the platform with further integrations and “even simpler workflows”.
Previously, Databricks has said one of the goals of MLflow was to allow users to deploy to multiple clouds. When it comes to Managed MLflow, there are two options, in the shape of AWS and Azure. Pricing for the standard Databricks service is the same on each, at $0.40 /DBU for Data Analytics. Azure also offers a Premium Plan which includes “Operational Security”.
Incidentally, Microsoft took part in Databricks’ most recent funding round. At the time, Databricks CEO Ali Ghodsi said Databricks would expand onto other clouds in time.
Part of Databricks’ pitch around MLflow is that data science and machine learning workflows could do with more discipline and reproducibility.
Databricks isn’t the only one beating this drum. Spell, founded by a former Facebook AI research director of engineering, launched earlier this year promising to give customers a “complete end-to-end system for exploring, training, building, automating and serving models built with deep learning” as well as access to more exotic hardware.