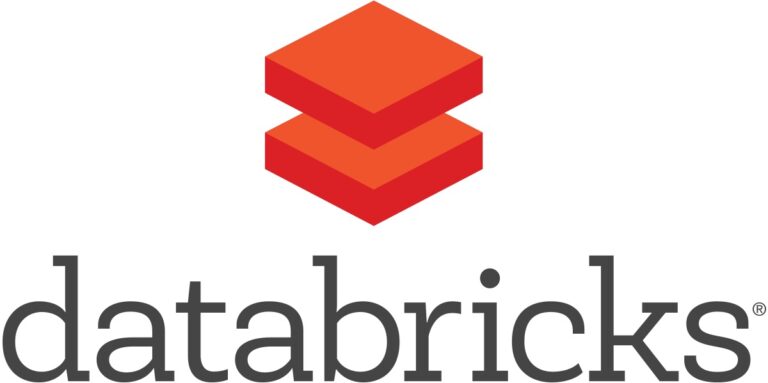
Databricks has announced support for Apache Spark 2.4.0, the open source cluster framework first developed by its own CTO, Matei Zaharia.
Spark 2.4.0 was released earlier this month, with key new features including Barrier Execution Mode to ease integration with deep learning frameworks, experimental Scala 2.12 support, and improved Kubernetes integration.
According to a blog post announcing Spark 2.4.0 Barrier Execution Mode is itself part of Project Hydrogen, an Apache Spark initiative to get round fundamental differences between big data and AI. As the developers put it, “Using this new execution mode, Spark launches all training tasks (e.g., MPI tasks) together and restarts all tasks in case of task failures.
Databricks said today Apache Spark 2.4.0 would be supported as part of its Databricks Runtime 5.0, which is itself released today. The company highlighted a new feature in the runtime, HorovodRunner, which it described as a simple way to “scale up deep learning training workloads from a single machine to large clusters, reducing overall programming and training time from hours to minutes.”
The new version also provides native integration with a range of popular deep learning frameworks, including TensorFlow, Keras and Horovod.
In a blogpost, Databricks product manager Todd Greenstein said the latest release delivered “substantional performance increases” in key areas, including a 16 per cent improvement in total execution time.