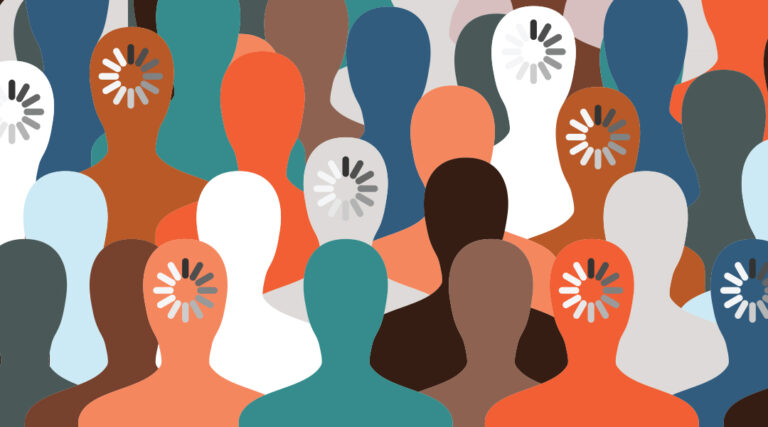
Facebook’s chief AI scientist Yann LeCun has been doing neural networks for quite a while now and has come to the conclusion that researchers are only as good as the hardware they have to work with.
While most consider themselves “abstract thinkers that aren’t limited by their hardware”, history suggests the opposite to be true . Therefore the type of hardware that is designed in the next decade will probably have a huge impact on where AI is going.
To make sure upcoming processor technology is fitted to the needs of the coming years, he used his keynote address at this year’s International Solid-state Circuits Conference to talk about his current paper in which he not only gives an overview of past deep learning hardware, but also takes a look into the future of machine learning approaches. And we’re all wondering what this will look like, right?
Looking at the market, most of today’s hardware is more of a dual-use affair, where learning activities of any kind often have to share the computational power with graphics processing. To get the most out of neural networks however, a purpose-built approach would be in order, if LeCun had his way.
A few architectural elements hardware designers should take into account at this stage are dynamic networks, associative memory, and sparse activations. Approaches that don’t use a fixed neural network architecture for example, but change the latter according to the input given (“Software 2.0” or “differentiable programming” as LeCun likes to call it), will make new kinds of hardware necessary, so that systems can run in the most efficient manner possible.
The associative memory mentioned could need similar support and is described by LeCun as a particular piece of a neural network, that serves as a memory structure a system can access at convenience. His example is a system that is able to “read” texts, memorise them, and answer questions about them later on.
Since artificial neural networks were once built to find a way to simulate the inner workings of a brain, there also seems to be a trend to carry this one further: while usually not all parts of the human brain are active at the same time, this is not true for large artificial neural nets. A way to save power for problems that need high computational power could therefore be to only activate a few units of a neural network at once.
According to LeCun, no one really knows how to do something like that at the moment, but since systems with low electrical power consumption and high computational power seem to be in demand, chances are good solutions will come up in the next five to ten years (a more efficient language could also help). And then hardware that could take advantage of sparse models would be very useful.
Researchers might also have to look into new ways of representing numbers, since regular arithmetics offer a kind of precision that might not really be needed for the kind of neural networks most products could use, LeCun feels.
That said, hardware developers shouldn’t feel restricted, since there are a number of opportunities for specialised approaches in AI. To train large machine learning systems for example, hardware with high computational power is needed. In those cases, cost doesn’t necessarily has to be an issue. Systems for training existing models can work with low precision arithmetics, so taking this into account could help to squeeze power consumption and make systems less expensive and therefore more accessible.
Embedded devices on the other hand could use some sort of neural network accelerator that is “low power, low cost” to be more useful, while the data centers where neural networks are run would need highly efficient systems with low latency and not too much hunger for electrical power.
According to the Financial Times, Facebook is already working on hardware addressing some of the issues mentioned – both on its own and in partnership with chip companies such as Intel (the only one confirmed yet, it seems). We’ll keep you updated on how Facebook’s quest to become one of the driving AI forces turns out.